The Two Sides of AI in Cybersecurity Businesses Must Know (Real-Life Use Cases Included)
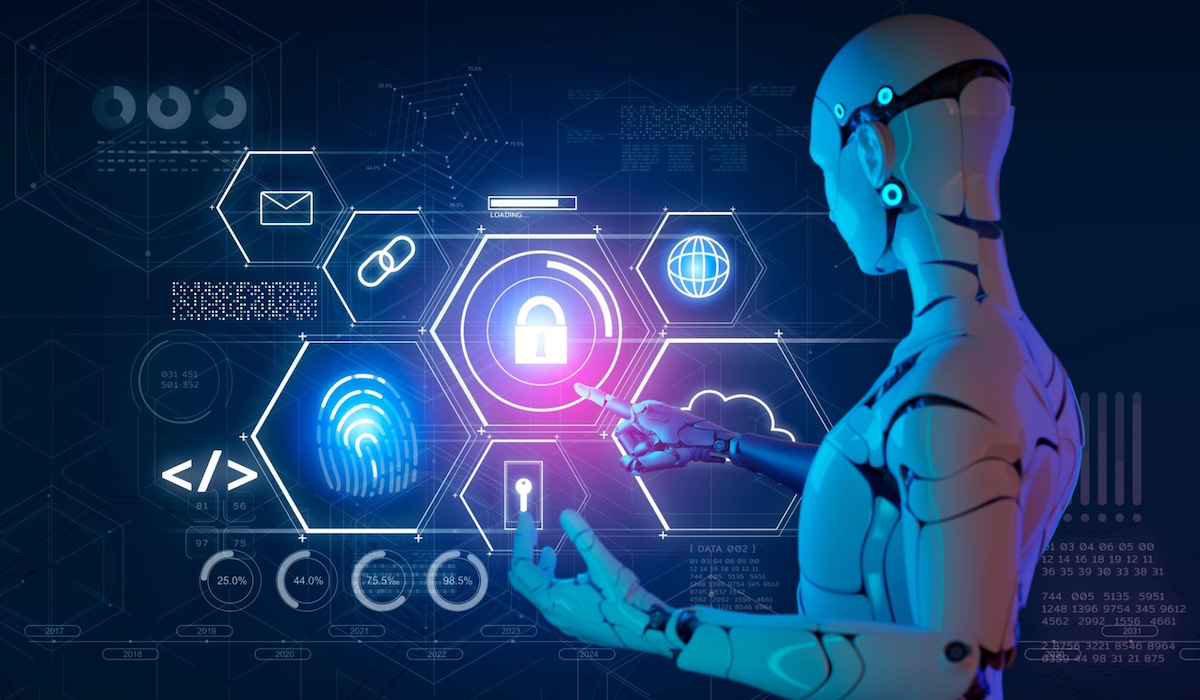
Businesses are more exposed to cyber criminals than before with the growing use of AI, especially generative AI, in their operations. And this is becoming more obvious with the recent incidents of cyberattacks. From advanced malware, and smart botnets, to personalized phishing emails and deep fake images and videos; hackers are launching AI-powered threats that are undetectable with traditional security tools and approaches.
It is more concerning as the speed and accuracy of these attacks have quadrupled with the use of AI in the cybersecurity field. On the other end, the technology enables businesses to shift from a traditional to a proactive security approach to strengthen their IT network and infrastructure. But that alone is not enough as there are several risks involved which can negatively affect their approach.
In this blog, we will highlight those risks, benefits they can expect from using AI, and the use cases.
What are the Benefits of Using AI in Cybersecurity?
1. Detecting Threats Faster and in Real-time
Threat detection is the first step for protecting businesses against cyber threats. However, traditional tools slow down the process exposing businesses in the hands of hackers. Using AI for threat detection reduces the risk as it goes beyond manual analysis such as pattern matching and threat hunting.
It also helps in detecting common, advanced, and evolving cyber threats which are hard to detect with traditional tools and approaches. With faster threat detection, the cybersecurity team gets ample time to mitigate the threats. According to IBM, AI can reduce the time to identify and respond to a threat by 14 weeks.
2. Preparing for Future Data Breaches
According to the recent report on data breaches worldwide, more than 8 billion records have been breached in the last one year. And among the top 10 industries, IT services and software were the most affected ones. Other industries like healthcare, manufacturing, telecoms, real estate, and cybersecurity were also on the list.
AI-based cybersecurity solutions are capable of preventing data breaches by identifying subtle anomalies that might indicate a possible breach attempt and security loopholes. They can also anticipate future attack vectors and tactics of hackers by analyzing the data, attack behavior, and patterns from past breaches.
By analyzing data breaches beforehand, security professionals get enough time to implement preventative measures before hackers even launch an attack. Not just external, using AI for network security protect businesses from threats and breaches emerging from the internal network and systems.
3. More Protection with Less Human Force
Cybercriminals are getting success in their goal because the world is facing a significant shortage of skilled security professionals. Around 3.5 million cybersecurity positions are vacant worldwide, as per Forbes. This gap makes businesses more vulnerable to cyberattacks as they don’t have enough expertise to defend their systems.
Artificial intelligence can fill this gap by augmenting the capabilities of human security professionals without increasing the need to hire a full-fledged security team. It can automate mundane tasks such as incident response, log analysis, security event monitoring, threat detection, and many more.
Automating these tasks frees up the existing security team of businesses for tasks where human judgment and expertise are required such as vulnerability assessment and threat detection.
4. Reduce False Positives and Alert Fatigue
Investigating and resolving security alerts is the biggest headache for security professionals. Increased alert overload not only distract them from focusing on genuine threats but also cause burn out among them. The impact- weak security and increased exposure to cyberattacks. Regulatory risk is another impact of alert fatigue as the security teams have less time to read and interpret the new cybersecurity regulations.
Using deep learning, a subfield of AI, in cybersecurity can reduce their workload and prevent businesses from both cyber and regulatory risks. It can help in the initial detection and flagging of the alerts saving time of security teams on reviewing the unnecessary alerts.
AI models working on deep learning, graph analysis, and anomaly detection techniques can help in accurately identifying real security alerts that need human intervention.
Train your employees to protect themselves and your business
Regular cybersecurity training programs organized by trusted software development companies can be implemented to educate employees about the latest cybersecurity risks and best practices, covering topics such as recognizing phishing attempts, creating strong passwords, and adhering to security policies.
Risks of AI in Cybersecurity
As said above, this technology is a double-edged sword there are a few risks involved. Businesses should be aware of those risks to prevent themselves from being the prey of hackers.
1. Bias Outputs
In cybersecurity, predictive analytics is very helpful to detect the potential threats. However , if the AI model is trained on bias, insufficient, or incorrect data then instead of accurate predictions, it may give false outcomes. For example, such systems can flag legitimate users as potential threats while giving access permission to unauthorized users. Therefore, it is important to assess the data and sources used to train the AI model for threat detection.
2. Data Poisoning
Manipulating training data, launching adversarial attacks, or poisoning algorithms are a few ways hackers use to trick AI systems. For example, hackers can inject fake samples in AI-based malware detection tools. As a result, they can misclassify the real malware as safe thus allowing hackers to easily launch malware into the systems.
3. Overreliance on AI
Relying completely on AI-based malware detection tools, threat detection systems, and other cyber solutions can put businesses at risk. It limits the critical thinking skills, and analysis power of human security professionals, and reduces the use of traditional security practices. This overreliance also give rise to new AI vulnerabilities, helping hackers to evade the security shield and steal business data easily.
Neural fuzzing- a technique to detect vulnerabilities in software security- is another way cybercriminals are using AI to launch cybersecurity attacks. Together with neural networks, they can learn the weak areas of the targeted software or system and use it to their advantage.
3 Real-Life Use Cases of AI in the Cybersecurity
AI can be good or bad, depending on who and how they use it. Businesses around the world are using AI for network security, threat detection, predictive analytics, malware detection, and more. Here are the real-life use cases.
Use Case 1. Fraud Prevention
More than 400 million people use PayPal for transactions. Manually analyzing each transaction to check for fraud activity is a task next to impossible. The US-based fintech major has adopted AI in its cybersecurity ecosystem to identify fraud transactions by capitalizing on its user behavior analysis capabilities.
Use Case 2. Phishing Detection
Tricking humans to click on suspicious links or installing malware is easy for hackers through personalized phishing attempts. AI can reduce such attempts by analyzing websites or emails, sender information, and files attached to the mail to classify whether it is legitimate or not.
Additionally, AI systems trained on machine learning algorithms are used to prevent users from disclosing confidential information by blocking trackers and indicating the threat level to the users. Google is a real-life example. It uses deep learning to filter phishing emails, spam, and emails with hard-to-detect images and hidden content.
Use Case 3. Preventing Zero-Day Attacks
Identifying zero-day attacks and new threats has always been the biggest challenge for security professionals. Unable to keep up with all the security patches and latest vulnerabilities is one of the reasons behind them.
AI-based cybersecurity systems help in both scenarios through behavioral analysis, heuristic analysis, and real-time anomaly detection. These systems continuously analyze how users are accessing the network, systems, and applications. If any unusual traffic or activity with no known signatures is observed, then it is marked as a potential threat.
Integrating with threat intelligence feeds, these AI systems provide the latest information on potential vulnerabilities and zero-day exploits. Darktrace is one of the real-life examples where the company uses machine learning algorithms to strengthen their zero trust security model and identify such exploits.
Final Words
The world is in the AI race where cybercriminals are the most active participants. They are experimenting and widely using AI to create highly intelligent viruses, advanced malware, and malicious code to target businesses. Businesses ignoring the security aspect and blindly implementing artificial intelligence tools and solutions in their tech ecosystem will have a drastic impact which would drag them years behind in this race. Therefore, it is important to mindfully use AI in cybersecurity to tackle both current and unexpected cyberattacks in the future.