Bridging the Gap: How RLHF Enhances AI Performance and Aligns with Human Values
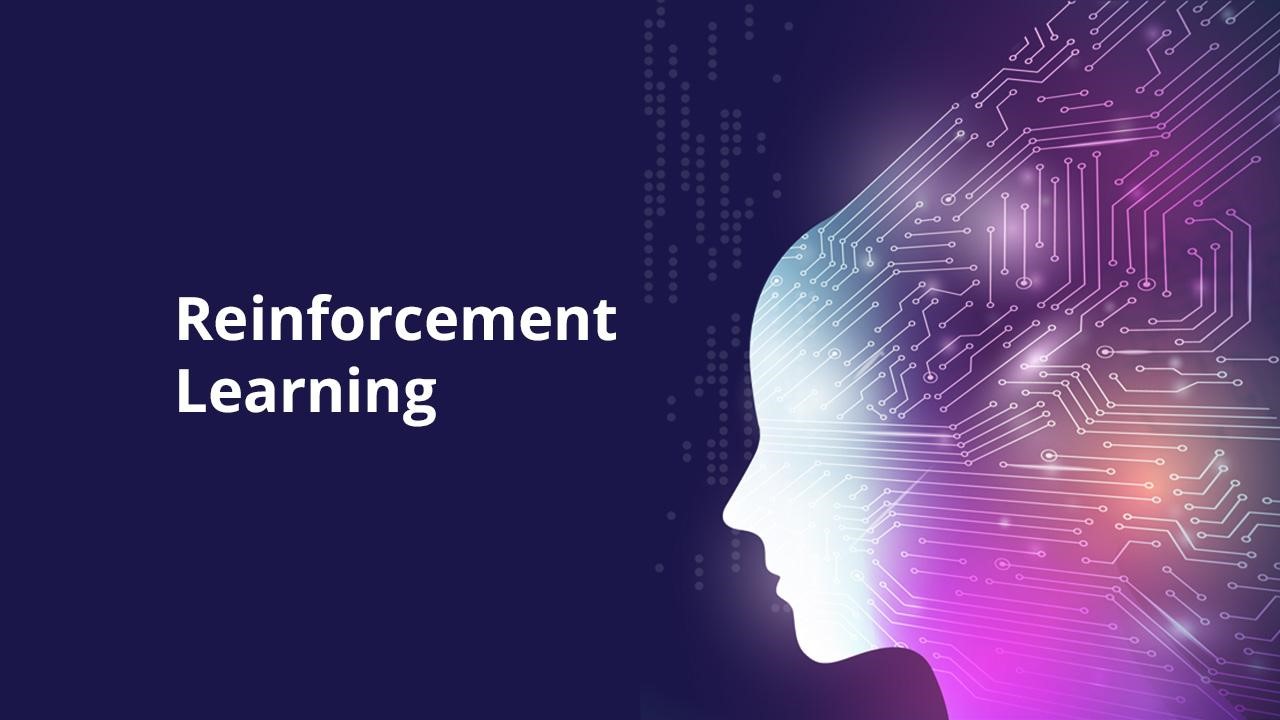
The world of AI technology is witnessing groundbreaking advancements, particularly in the realm of generative AI. These innovative developments can potentially change the working styles of industries and transform how we live and work. Reinforcement Learning from Human Feedback (RLHF) is an essential breakthrough that ensures AI models are in harmony with human values, providing helpful, honest, and secure responses.
Incorporating an efficient human feedback loop is crucial to leveraging the full potential of generative AI while mitigating risks. RLHF is a powerful machine learning approach that combines human insights with reinforcement learning to fine-tune AI models. AI models can better align with human values by integrating human feedback into training, leading to more accurate and reliable outputs. RLHF can enhance the learning process by capitalizing on human expertise. As humans assess and provide feedback on the model’s output, they can identify and correct any inappropriate, biased, or harmful responses, ensuring the model learns faster and more accurately.
Moreover, RLHF addresses the challenge of sample inefficiency in reinforcement learning, allowing AI models to learn more effectively and reducing the number of iterations required to master a task. By integrating RLHF into AI development, businesses and researchers can unlock the full potential of generative AI, leading to unparalleled innovation and progress across various sectors. Dive into the fascinating world of RLHF and discover how it can shape the future of AI technology.
What is reinforcement learning?
Reinforcement learning (RL) is a machine learning technique where agents learn to make optimal decisions by receiving feedback as rewards or penalties. Two main types of reinforcement are positive and negative reinforcement. Positive reinforcement strengthens behavior through rewards, while negative reinforcement strengthens behavior by removing or avoiding negative stimuli. This learning process is akin to training animals or how infants learn by interacting with their environment. In RL, the agent, such as a game player or autonomous vehicle, engages with its environment, constituting the “world” in which the agent operates.
Two essential components of RL are the agent and its environment, which interact via an action space and a state (observation) space. Actions can be discrete or continuous, and states convey information about the environment, with observations providing partial descriptions of states.
A vital aspect of RL is the reward system. Agents are trained to make the best decisions by receiving positive, negative, or neutral rewards based on their actions’ effectiveness in achieving specific goals. The reward function is critical to a model’s success, and balancing short-term and long-term rewards using a discount factor (gamma) is essential.
Another key concept in RL is balancing exploration and exploitation. It is crucial to encourage agents to explore their environment rather than solely relying on existing knowledge. This is done by implementing an additional parameter (epsilon) that dictates the percentage of situations in which the agent should take a random action (explore).
Various algorithms are employed in RL, including Q-learning, SARSA, and Deep Q-Networks (DQN). Q-learning and SARSA are value-based algorithms that update the value function of state-action pairs, whereas DQN uses a neural network to approximate the Q-value function.
RL is applicable in various fields like gaming, robotics, and autonomous vehicles, offering potential solutions to complex decision-making challenges in dynamic environments that are difficult to address using conventional programming methods.
What is reinforcement learning from human feedback?
Reinforcement Learning from Human Feedback (RLHF) is an approach that enhances traditional reinforcement learning (RL) by incorporating feedback from human supervisors. This method addresses the limitations of automated reward systems, which may struggle to capture subjective aspects of certain tasks.
In conventional RL, a mathematical function defines the reward signal based on the task’s goal. However, this may not fully account for human-centric factors, such as the subjective taste of a pizza cooked by a robot. RLHF integrates human feedback to consider these subjective aspects, resulting in a more comprehensive reward signal.
Since relying solely on human feedback can be time-consuming and costly, RLHF typically combines automated and human-provided reward signals. The primary feedback comes from the automated reward system, while human supervisors supplement it with additional input. This can involve occasional rewards or punishments or providing data to enhance the automated reward signal through a reward model.
RLHF offers several advantages, including improved safety and reliability of RL agents, as humans can intervene and provide feedback when the agent performs poorly or makes mistakes. Furthermore, RLHF ensures that the agent’s learning aligns with human preferences and values, leading to a more satisfactory performance of tasks.
How does RLHF work?
In reinforcement learning, an agent learns to interact with an environment, gaining experiences to maximize cumulative rewards. In NLP tasks, environments are datasets or annotated texts, and agents can be machine learning models like language models, decision trees, or neural networks.
Reinforcement Learning from Human Feedback (RLHF) is a versatile, multi-stage process that can be applied to NLP tasks and other domains, such as robotics, gaming, or advertising. In these cases, agents could be robots, game players, or ad systems, with human feedback sourced from users, experts, or crowdsourcing platforms.
Reinforcement Learning from Human Feedback (RLHF) is a process that refines AI models by incorporating human preferences into their learning mechanism. The method involves three main steps: pretraining language models, generating data to train a reward model, and optimizing the original language model with reinforcement learning.
- Pretraining language models: Large-scale language models are pretrained on vast amounts of text data using unsupervised learning techniques, such as autoencoding or predicting masked tokens. This allows the model to understand language structure and generate coherent, semantically meaningful text. Transformer-based architectures, such as GPT, BERT, and RoBERTa, are commonly used for pretraining language models.
- Generating data to train a reward model: A reward model (RM) is created to integrate human preferences into the RLHF system. The RM assigns a score reflecting human perception of the generated text. Human annotators are involved in ranking the generated text outputs, which helps create a better-regularized dataset. Training the RM can be done using fine-tuned language models or training them from scratch on preference data.
- Optimizing the original language model with reinforcement learning: With a functioning initial language model that generates text and a preference model that assigns scores, reinforcement learning is used to fine-tune the language model concerning the reward model. The Proximal Policy Optimization (PPO) algorithm is employed for fine-tuning, ensuring the model generates coherent text snippets while maintaining its initial learning.
The RLHF process can continue by iteratively updating the policy and reward model together. As the RL policy updates, users can keep ranking the outputs against the model’s earlier versions, refining the AI model over time.
Red teaming is an essential aspect of the RLHF process, where human evaluators with expertise in different fields assess AI models, identifying gaps and recommending improvements. These evaluators test models in various scenarios, including edge cases and unforeseen circumstances, to identify limitations and vulnerabilities that need to be addressed. By testing the models in challenging situations, red teaming helps ensure the robustness and safety of the AI systems.
Final Thoughts
Reinforcement learning from human feedback plays a pivotal role in enhancing the precision and dependability of AI models. By factoring in human input, these models can better align with human expectations and values, improving user experiences and greater confidence in AI systems.
In the context of generative AI models, RLHF is crucial. Left to their own devices, such models may generate erratic, incongruous, or offensive outputs, potentially eroding public trust in AI. With human reinforcement integrated into the training process, generative AI models can produce results that align with human preferences, standards, and values.
One significant application of RLHF is in chatbots and customer service. By employing RLHF in training chatbots, businesses can ensure their AI-driven customer service can accurately comprehend and address customer inquiries, providing an enhanced user experience. Moreover, RLHF can improve the precision and dependability of AI-generated visuals, text captions, financial trading decisions, and even medical diagnoses, emphasizing its crucial role in developing and deploying AI technology.
As AI technology advances and diversifies, it is essential to prioritize developing and implementing RLHF to guarantee the long-term success and sustainability of generative AI systems.