Augmenting Intelligence: The Role of Generative AI in Data Engineering and Its Impact
The turn of the decade is seeing a massive generation and influx of data – thanks to the numerous systems companies seem to be adopting these days. Most Fortune 500s organizations are grappling with the challenges with data homogeneity and the need for real-time insights.
The twin synergies of data engineering and artificial intelligence (AI) is emerging as a critical enabler for operational efficiency and simplifying IT architectures.
Keep scrolling as we explore the challenges of data deluge and the advantages of utilizing Generative AI services in data engineering.
Why do we need the confluence of AI and Data Engineering in 2024?
Data engineering lays the foundation for AI by making data accessible, reliable, and analysis-ready. AI, on the other hand, builds models on this data to generate insights, predictions, and automations. Together, they form a potent combination – Augmented Intelligence, to transforms raw numbers into actionable intelligence.
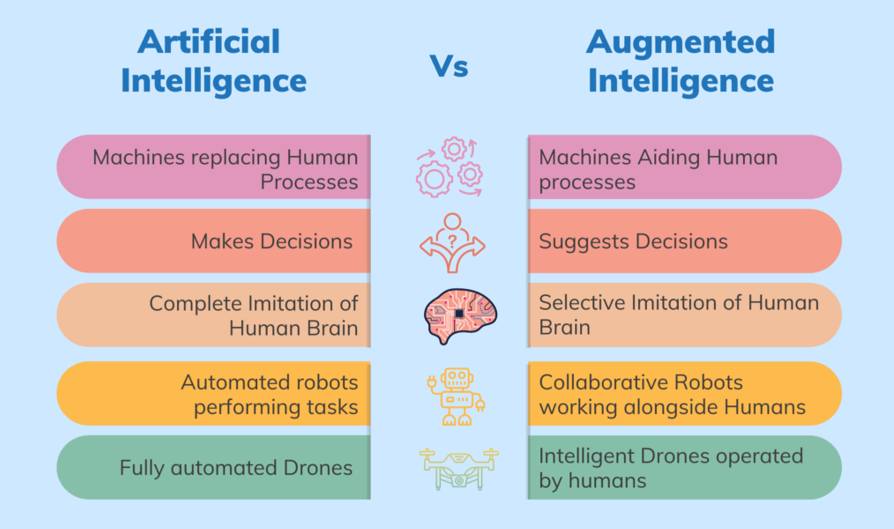
Augmented Intelligence isn’t another tech mumbo-jumbo, here’s why AI and Data Engineering are less likely to work separately in 2024:
- Data Volume and Complexity
-
- Data generated is projected to reach 175 zettabytes by 2025, poses significant challenges in storage, management, and processing.
- Diverse data sources and formats complicate data integration and harmonization.
- Real-Time Processing Needs
- Businesses demand real-time analytics to make immediate decisions, requiring robust data pipelines and low-latency processing.
- Data Quality and Governance
- Ensuring data accuracy, consistency, and compliance with regulations like GDPR is paramount but challenging.
What solutions are offered by Augmented Intelligence?
Ever since ChatGPT came into the picture with the backing of OpenAI and Microsoft, departments across are squabbling to squeeze every drop of productivity assist from GenAI. Data Engineers are putting it to brilliant use:
The enhanced use-cases of Data Engineering and AI in tandem are simplifying the data architectures in orgs:
- Scalable Data Infrastructure
- Modern data engineering services utilize scalable cloud-based solutions like AWS, Azure, and Google Cloud Platform to handle large data volumes efficiently.
- Technologies such as Apache Kafka and Spark facilitate real-time data streaming and processing.
- Data Quality Management
- AI algorithms enhance data quality by identifying and rectifying anomalies, duplications, and inconsistencies automatically.
- Machine learning models predict and fill gaps in datasets, improving overall data reliability.
- Generative AI in Data Engineering
- Generative AI can automate the creation of synthetic data, aiding in testing and training AI models without compromising privacy or security.
- For instance, generative AI for data engineering can simulate customer interactions to improve service delivery and personalize user experiences.
Benefits of Integrating AI and Data Engineering
- Enhanced Decision-Making
- AI models simplify vast datasets to assist CXOs have the most relevant insights on their fingertips – leading to faster and quantitative decision making.
- Predictive analytics enable proactive decision-making, reducing risks and capitalizing on opportunities.
- Operational Efficiency
- Automation of routine data engineering tasks, such as ETL (Extract, Transform, Load) processes, frees up human resources for more complex problem-solving activities.
- AI-driven analytics streamline supply chain management, optimizing inventory levels and reducing operational costs.
- Personalized Customer Experiences
- AI-powered data engineering services facilitate the creation of 360-degree customer views, enabling personalized marketing and improved customer service.
- Companies like Netflix and Amazon use AI to analyze viewing and purchasing patterns, respectively, delivering tailored recommendations and enhancing user engagement.
Future Trends and Considerations
- Ethical AI and Data Privacy
- As AI becomes more integrated into data engineering, ensuring ethical use and data privacy will be crucial. Implementing robust data governance frameworks is essential to maintain trust and compliance.
- Augmented Intelligence
- We’ve already seen steps taken by GenAI in Data Engineering, AI can handle data-intensive tasks, while humans focus on strategic thinking and creativity.
- Generative AI Use Cases in Data Engineering
- Emerging use cases for generative AI include advanced natural language processing for automated report generation and enhanced data visualization techniques, making complex data accessible to non-technical stakeholders.
How do companies stay ahead of the curve?
The synergy between AI and data engineering is now more of a strategic imperative. Tested and trusted players like Polestar Solutions help Fortune 500s addresses the pressing challenges with innovative solutions to help businesses unlock unprecedented Data Engineering hacks, from enhanced decision-making to operational efficiencies and personalized customer experiences. As we move forward, embracing the ethical and augmented intelligence paradigms will be key to sustaining competitive advantage and driving long-term success.