5 tools that will help you succeed as a data scientist
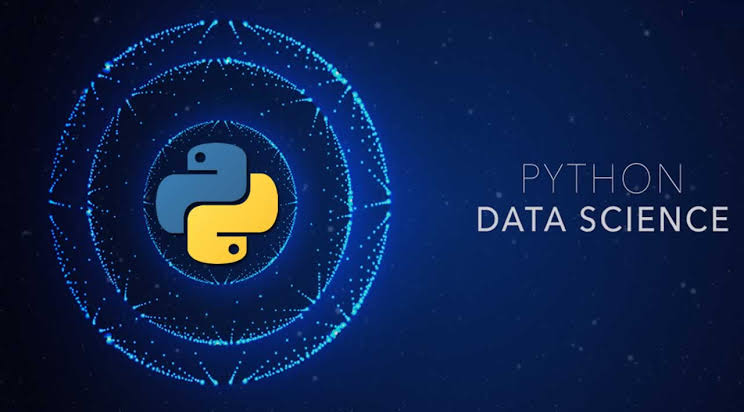
Data science education lets you inside one of the most interesting and opportune professional fields, unless of course, you would rather be on a path of data scientific wisdom working as a researcher. The demand for able minds in the applied data science sector has been consistently increasing over the past decade. Even in this pandemic ridden situation the industry has not stopped growing. But somehow, a lot of people with data science degrees are unable to find suitable employment. A good percentage of data science professionals working in different capacities want to change jobs. This sort of situation is not very uncommon but that should not stop you from getting what you deserve and and more importantly what you think is best for you.
Some bugs that need to be addressed first
Oftentimes it occurs that a professional holding a data science degree does not fit into a company because of a technical gap. One can never stress enough on the importance of industry relevance in terms of acquiring new skills or attending courses. There is another serious bug which needs to be fixed. Young aspirants often skip steps while preparing for the role of a data scientist. What they need to realize is that they are not going to be hired straight away in the capacity of a data scientist, if they are it would be a sham. So, here is a list of 5 tools in an increasing order of importance for a data science role.
Hadoop skills
While the word around the internet is that Hadoop is almost obsolete thanks to cloud computing and advanced analytics practices powered by machine learning, the reality is somewhat different. In this regard you should read more about why companies are using Hadoop with Python. The Hadoop distributed file system or HDFS which played a huge part in democratizing big data, is still largely in use across various industries. Tools from the Hadoop software suit are also used for data mining. Spark, another great tool for data processing runs well on Hadoop.
Hadoop skills can be a good way to enter the data science workforce – a strong foundation for acquiring more advanced skills.
Data wrangling skills
While data has the potential to drive important business decisions, most of the data available to businesses is unstructured. This unstructured data, in the form of images, videos, and audio, can be used for drawing useful insights but before that it needs to be cleaned, and organized. Data wrangling refers to the tools and procedures applied to clean data and organize it in a certain structure so that the data can be sent through a predictive model.
Data wrangling skills make a data science professional self-sufficient. A large number of data scientist roles consist of these responsibilities. These skills increase your employability manifold.
Data visualization skills
The data scientists’ work is only half done until the results are expressed in a comprehensible manner to the stakeholders. In fact, data visualization aids in the interpretation of data as well. Tools like Tableau used for data visualization can come very handy for data science professionals. In fact a lot of companies include Tableau skills in the list of requirements for data science roles. These skills help data science professionals communicate in a meaningful way.
R skills
R is among the few tools that have been used by data professionals for decades with great consistency. It is an open source tool that allows data scientists to program statistical analysis models. It has inbuilt functions for computing, and it also offers a great flexibility in terms of data sources. R also comes with interesting data visualization options.
Python skills
Coding is an essential part of a data scientist’s job description. While as a data scientist you can always count on the software engineers to code your vision into reality, you often need to give them a model to work with. Python is one of the best tools for data science professionals to create models. Personnel with Python data science skills are enjoying ever increasing demand across industries.
Python has a bunch of inbuilt libraries that allow you to program predictive models, and machine learning algorithms with comparative ease. Knowing your way around Python can make a real difference in your career
While the data science landscape is characterized by change these tools have stayed relevant for a long time through constant optimization according to industry standards. Learn these tools and stay alert for new tools that pop up and show great potential.