What Is Predictive Coding in eDiscovery
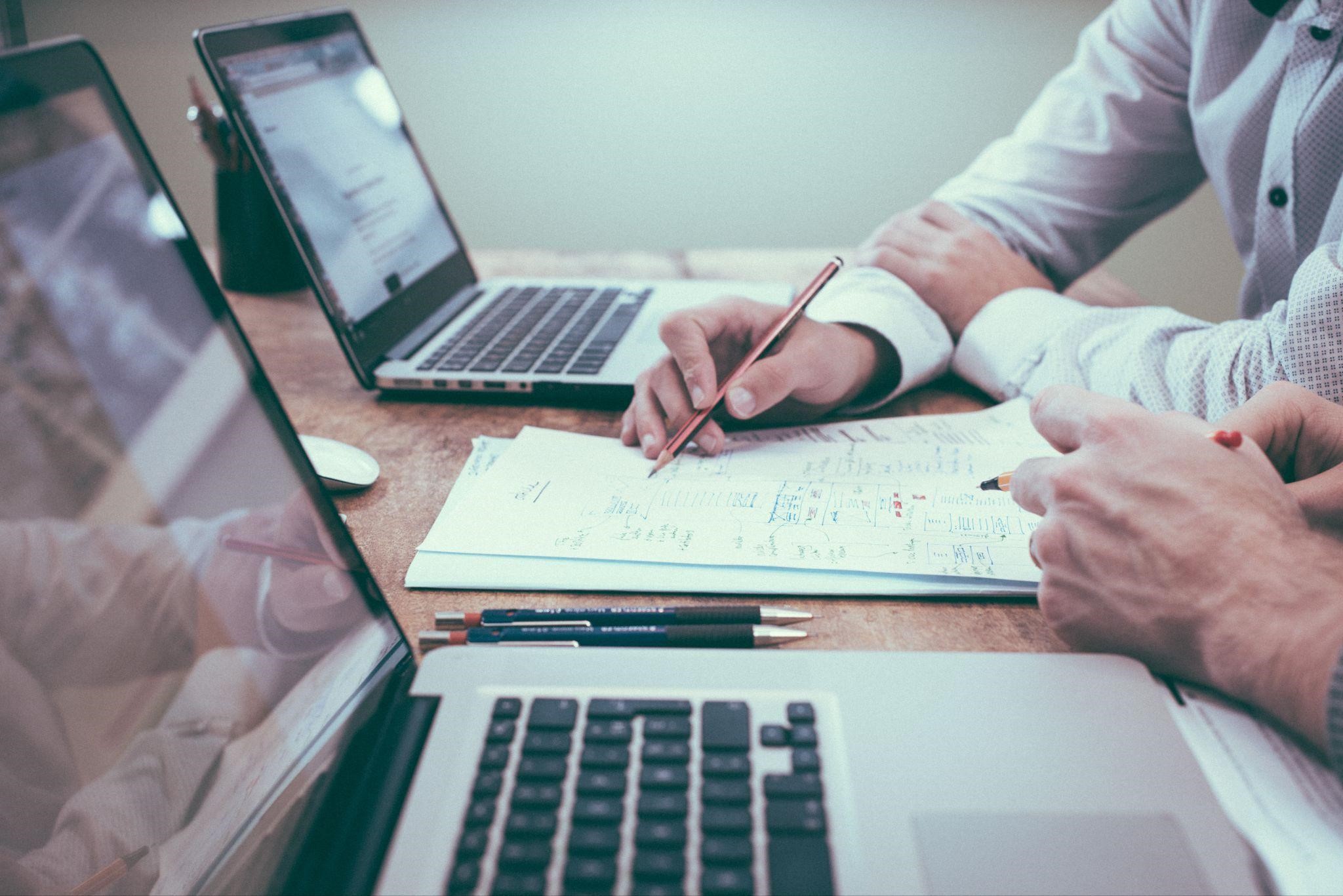
Artificial intelligence has become a part of every aspect of society.
The legal aspect is also affected through the implementation of predictive coding and eDiscovery.
But what is predictive coding in eDiscovery, and how do these terms correlate?
This article will cover the aspects of predictive coding theory. It will also provide a predictive coding eDiscovery definition.
By the end, you will have a complete overview and understanding of this type of legal document research.
Image credit: Scott Graham/Unsplash.com
What Is Predictive Coding in eDiscovery?
Predictive coding is the technique of leveraging machine learning. Its goal is to search and find relevant documents based on previous review patterns. In eDiscovery, predictive coding finds ESI (electronically stored information) for legal purposes.
It is wrong to assume that predictive coding is another name for the algorithm used to extract information. It is, in fact, the entire process, which also includes the algorithm.
The basic process of predictive coding
Predictive coding is a type of machine learning.
As a process, it is relatively complicated to explain. The most basic way to provide an explanation of predictive coding in electronic discovery is by understanding how it works.
Predictive coding begins when the dedicated software learns specific search patterns. It does that through seed set data.
This seed set data can be a simple reference document. Humans have set the document as an example of what the machine should look for.
Skilled human reviewers then mark documents as relevant or irrelevant to a case. This tells the machine to use only the relevant documents during the learning phase.
As the predictive coding process progresses, the machine improves. It produces quicker, more accurate results.
When Was Predictive Coding First Used for eDiscovery
Machine learning, data analytics, and information retrieval have existed for many years. In fact, the term machine learning was first used by Arthur Samuel in 1959.
However, predictive coding and machine learning tools were not used for legal proceedings before 2012.
In 2012, a state court ruling was issued by the state judge in Virginia. It allowed predictive coding in eDiscovery examples, like the Global Aerospace case.
Since then, the use of predictive coding has drastically increased. Judges have become more willing to approve the use of this technology. They often favor it due to the time-saving and cost efficiencies it offers.
How Predictive Coding Compares to CAL and TAR?
CAL stands for continuous active learning. It is a process used for machine learning but in a different way than predictive coding.
While predictive coding starts with a seed data set, CAL runs continuously. It is an automated approach. It begins to learn as soon as the team of human reviewers starts to sort relevant from irrelevant pieces of information.
TAR (technology-assisted review), on the other hand, is used to represent different ways that a machine can learn to sort and review documents.
Both predictive coding and continuous active learning are approach types to a technology-assisted review. They can be used to search, find, and review legal documents.
If these two approaches are similar, why would a team of reviewers use predictive coding for eDiscovery?
Let’s have a look at the benefits that predictive coding can offer.
Benefits of Predictive Coding
Predictive coding offers multiple functionality advantages compared to the old-fashioned human document sifting.
These advantages depend on each case.
It is less expensive to implement
From a financial aspect, using the predictive coding technique saves money compared to a human document revision.
Some cases may have thousands of documents that need reviewing, meaning that more expert people are needed to review them in a short amount of time. Conversely, this means an automatic increase in costs.
On the other hand, using predictive coding is much cheaper, as the process starts to rely on automation and is supervised by only a few human experts.
It does the heavy lifting
Using predictive coding for eDiscovery means machines do all the heavy lifting regarding data processing and review.
All that human experts need to do is supervise the process and ensure the proper documents are being reviewed.
It is less susceptible to mistakes
Going through thousands of documents for a case can be exhausting for humans. There is a high chance that someone, somewhere, will miss some part of vital information.
Machines rarely make mistakes and miss essential information if appropriately adjusted. More importantly, they can even uncover relevant information semantically linked to the case.
This means they understand the case and try to extract as much relevant information as possible.
It is quick and efficient
One of the most significant advantages of the predictive coding technique is its efficiency and swiftness in researching and finding relevant information.
This offers several advantages on its own.
For one, the faster the information is researched, the quicker the human counsel will learn all the relevant facts about the case. This means less preparation time and a better strategy in court.
Second, the quicker predictive coding uncovers relevant documents, the lesser the litigation costs. This also means the case will be solved quicker, saving time for the clients.
Reasons Why Legal Teams Avoid Predictive Coding
Besides some of the benefits that we’ve just analyzed, there are also some challenges that legal teams face when using predictive coding.
It is difficult to set up
Predictive coding, as a type of machine learning, is not yet capable of performing tasks independently from the start. It must be set up properly by highly qualified experts in the field of law.
This means that the entire process starts and ends with human supervisors. They must be competent enough to gather the required seed set of data, set up the algorithm, and check that it’s working correctly.
Only this approach can ensure that the data collected by predictive coding is accurate and semantically connected.
It doesn’t encourage 100% reliability
Even though it’s starting to be widely used by legal teams, predictive coding is still not a 100% reliable solution.
This is because machine learning is still raising questions, such as how the algorithm can be capable of understanding semantically related topics and extracting them.
There is no certain answer to this type of question since the algorithm is self-taught.
On top of that, the opposing counsel may raise concerns about the training methods of the algorithm.
It promotes uncertainty among legal teams
Another challenge with predictive coding in eDiscovery is that lawyers and legal teams are still unfamiliar with its process.
They are intimidated by the level of involvement it takes to master predictive coding, as well as the amount of dedication it would take to implement it.
Because of this, a large part of the legal community is still hesitant about predictive coding for electronic evidence searches.
Conclusion
There is no doubt that artificial intelligence will continue to evolve in the future.
For the time being, predictive coding has proven to be a reliable asset and method in eDiscovery.
We hope that you now know what is predictive coding in eDiscovery and that you will see it as a reliable tool that can save both time and money during the electronic discovery process.